In today’s rapidly evolving technological landscape, data annotation tech has emerged as a pivotal element in the development of artificial intelligence (AI) and machine learning (ML) systems.
Whether it’s for self-driving cars, voice recognition software, or advanced medical imaging, machine learning models rely on vast amounts of accurately labeled data to function efficiently.
This article explores the significance of data annotation tech, its various applications, and how businesses can leverage it for better AI results.
What is Data Annotation Tech?
Data annotation tech refers to the process of labeling or tagging data such as images, text, or audio to provide context and meaning that machines can understand. In essence, it is the groundwork that allows machine learning algorithms to make sense of raw data.
Without data annotation, AI models would be unable to differentiate between objects, sounds, or contexts, limiting their potential.
For example, in image recognition, data annotation involves labeling images with categories such as “dog,” “cat,” or “car,” allowing an AI system to recognize and classify these images accordingly.
Similarly, in natural language processing (NLP), data annotation helps label text for sentiment analysis, keyword extraction, and entity recognition.
Read more: Metro by T Mobile
Types of Data Annotation
There are several types of data annotation tech, and each serves a different purpose based on the type of data being labeled:
- Image Annotation: This involves tagging images with metadata, such as bounding boxes, segmentation masks, or points of interest. It is widely used in autonomous vehicles for object detection or medical imaging for identifying tumors.
- Text Annotation: This is the process of adding labels or tags to text data. It includes tasks such as sentiment labeling, part-of-speech tagging, and named entity recognition. Text annotation is crucial in training chatbots and voice assistants.
- Audio Annotation: In this process, audio data is transcribed or labeled to help systems understand speech or other sounds. It is essential in voice recognition technology and for creating accurate subtitles for videos.
- Video Annotation: Video annotation combines multiple types of labeling, such as object tracking across frames, scene segmentation, and action recognition. It is particularly useful in surveillance, sports analysis, and autonomous driving.
Applications of Data Annotation Tech
The impact of data annotation tech is felt across a wide variety of industries. Here’s how it’s transforming several key sectors:
1. Autonomous Vehicles
In the development of self driving cars, data annotation is crucial for object detection and understanding road environments. AI systems need labeled data from cameras and sensors to identify pedestrians, road signs, and other vehicles. Proper data annotation enables these systems to make real-time decisions to ensure safety.
2. Healthcare
Data annotation plays a vital role in healthcare by improving diagnostic accuracy. Annotating medical images like X-rays, CT scans, and MRIs allows AI models to identify diseases such as cancer, detect abnormalities, and even recommend treatment plans. By labeling images with accurate annotations, data annotation tech helps doctors make faster and more informed decisions.
3. E-Commerce
For e-commerce platforms, data annotation enhances search functionalities and product recommendations. By tagging product images with attributes like color, size, and category, machine learning models can help customers find exactly what they are looking for. Text annotation also enables AI driven reviews and feedback analysis, improving customer service.
4. Finance
In the financial sector, data annotation tech is used for fraud detection, sentiment analysis, and algorithmic trading. By annotating transactional data or historical market trends, machine learning models can predict market behaviors, identify fraudulent transactions, and optimize investment strategies.
5. Security and Surveillance
AI systems rely on data annotation to analyze video feeds and detect suspicious activities. By annotating images and videos with tags such as intruder, vehicle,” or “weapon,” security systems can quickly alert authorities to potential threats, enhancing safety in public spaces.
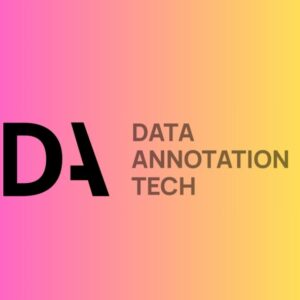
Benefits of Data Annotation Tech
The rise of data annotation tech has revolutionized how AI systems are trained, offering several advantages:
1. Improved Accuracy
With precise and accurate labeling, machine learning models become significantly more accurate. The more annotated data AI systems are fed, the better they can identify patterns and make informed decisions. This results in higher-quality outcomes, whether it’s object detection, sentiment analysis, or any other machine learning task.
2. Efficient AI Training
Data annotation serves as the foundation of efficient AI model training. With annotated data, algorithms can quickly learn from labeled examples and generalize their understanding to new, unseen data. This reduces training time and enhances model performance.
3. Customization
Businesses can use data annotation tech to customize AI models to their specific needs. Whether it’s a niche industry or a particular use case, data annotation can ensure the AI system understands and processes data in a way that aligns with the organization’s goals.
4. Scalability
As businesses scale, the need for vast amounts of labeled data grows. Data annotation tech can be scaled to handle enormous datasets, ensuring that businesses can continue training AI models effectively as they expand and diversify.
Challenges in Data Annotation
While data annotation tech offers significant benefits, there are also challenges involved in the process:
1. Time-Consuming
Data annotation can be a time-intensive task, especially when dealing with large datasets. Annotating thousands or millions of images, texts, or audio files requires careful attention to detail, which can be resource-heavy.
2. Cost
Hiring skilled annotators or utilizing third-party services to annotate data can be expensive, particularly for large-scale projects. For businesses with tight budgets, this can be a significant barrier to entry.
3. Quality Control
Ensuring the accuracy of annotations is critical. Inconsistent or incorrect labeling can lead to inaccurate AI models. It’s essential to have rigorous quality control measures in place to avoid errors that can affect the performance of machine learning models.
The Future of Data Annotation Tech
As AI continues to grow and evolve, so will data annotation tech. The future promises innovations such as:
1. Automated Data Annotation
AI itself may assist in automating the annotation process, making it faster and more cost-effective. While human annotators will still be necessary for complex tasks, AI tools can help speed up simple labeling tasks and reduce the burden on human resources.
2. Crowdsourced Annotation
With the rise of crowdsourcing platforms, businesses can tap into a global pool of annotators. This allows them to scale data annotation efforts quickly and affordably, while ensuring a diverse range of perspectives and input.
3. Enhanced AI Integration
As machine learning models become more advanced, data annotation tools will become more integrated with AI systems, allowing for more seamless data labeling and quicker iteration cycles.
Final Thoughts
The importance of data annotation tech in AI and machine learning cannot be overstated. As industries continue to adopt AI-powered solutions, the demand for high-quality annotated data will only increase. Whether it’s improving accuracy, enhancing model training, or enabling specific use cases, data annotation serves as the foundation of intelligent systems.
By understanding its importance and addressing its challenges, businesses can harness the full potential of data annotation tech and drive the next wave of innovation in AI. By incorporating data annotation into your AI initiatives, you’re not just labeling data—you’re preparing for a future where intelligent systems can perform with greater precision and efficiency, transforming the world as we know it.